Quantum Data Science Team is in the top 20 in the 1st Agriculture-Vision Challenge
Quantum team participated in the first Agriculture-Vision Challenge in April 2020 and ended up being in the top 20 list.
The Challenge was conducted by IEEE/CVF CVPR, and was aimed to encourage research in developing novel and effective algorithms for agricultural pattern recognition from aerial images.
“Being a company that possesses deep expertise in data science within AgriTech domain, we were pleased to be invited to take part in the challenge and compete with international teams.
The most challenging part was that we had limited time to showcase all our expertise, at the same time this encouraged us to apply unique approaches and come up with new ways to solve pressing problems in agriculture”, says Mike Yushсhuk, Head of Data Science in Quantum
Such areas as aerospace and medicine have already benefited and successfully utilize computer vision every day in their domains. Computer vision in agriculture is gaining more and more attention to the growth of deep learning implementation to solve various tasks within the industry. There have been numerous efforts to apply pattern recognition techniques in agriculture to increase potential yield as well as prevent losses.
57 participating teams from all over the world tried to recognize field patterns, such as cloud shadow, double plant, planter skip, standing water, waterway and weed cluster from 21,061 aerial and multi-spectral farmland images captured throughout 2019 across the US via semantic segmentation paradigm.
Quantum team comprised of 3 people – two data scientists and a team lead. The team had conducted 80 experiments within the 3 weeks of participation, where they have tried various loss functions for semantic segmentation, 5 different modifications of neural networks, and several pre and post-processing options. While solving the task, Quantum team has come up with its own enhancement of ResNet architecture.
All the above had brought Quantum to the list of top 20 participants with the most innovative models.
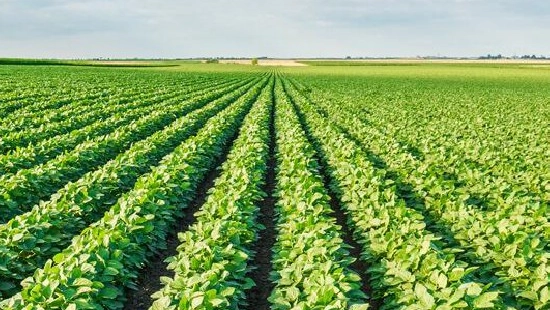
About the IEEE Computer Society
The IEEE Computer Society is the premier source for information, inspiration, and collaboration in computer science and engineering. Connecting members worldwide, the Computer Society empowers the people who advance technology by delivering tools for individuals at all stages of their professional careers. Our trusted resources include international conferences, peer-reviewed publications, a robust digital library, globally recognized standards, and continuous learning opportunities.
About Quantum
Quantum is a data science and software engineering company with international presence and R&D centers in Ukraine. We serve large software companies and quickly-growing startups all over the world, investing in our clients’ competitive advantages and capacity using cutting-edge technologies.